What You Need to Know Before
You Start
Starts 25 June 2025 06:41
Ends 25 June 2025
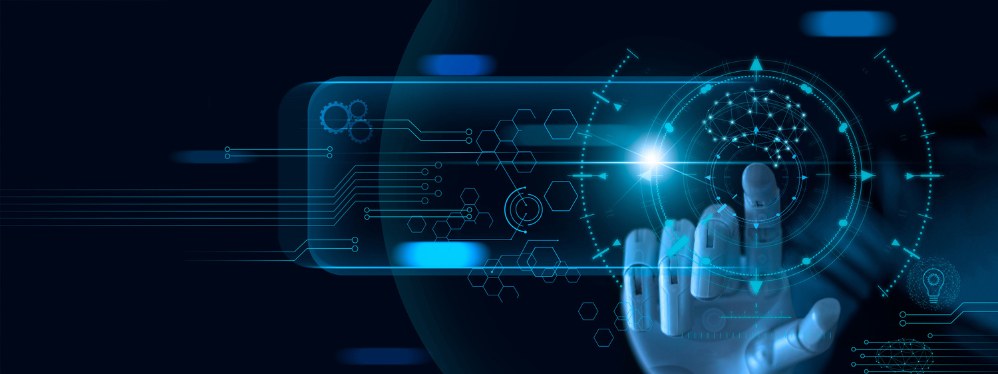
Enhancing Traditional ML with Generative AI
659 Courses
27 minutes
Optional upgrade avallable
Not Specified
Progress at your own speed
Free Trial Available
Optional upgrade avallable
Overview
As AI evolves, professionals are challenged with understanding how emerging Generative AI capabilities can coexist with traditional machine learning approaches. In this course, Enhancing Traditional ML with Generative AI, you’ll learn when to use these technologies and how they compliment each other.
First, you’ll explore the differences and synergies between traditional AI and genAI. Next, you’ll discover how genAI accelerates traditional AI .
Finally, you’ll learn how to apply AI assisted development. When you’re finished with this course, you’ll have the skills and knowledge of enhancing traditional ML with generative AI needed to use these technologies together.
Syllabus
- Introduction to AI and ML
- Understanding Generative AI
- Differences Between Traditional ML and Generative AI
- Synergies Between Traditional ML and Generative AI
- How Generative AI Accelerates Traditional ML
- Applying Generative AI in Traditional ML Development
- AI-Assisted Development
- Future Trends and Challenges
- Conclusion and Next Steps
Taught by
Jillian Kaplan
Subjects
Computer Science